Zero Defect Spindle Factory Twin
GF Trial: Zero Defect Spindle factory Powered by big data and AI across manufacturing lifecycle
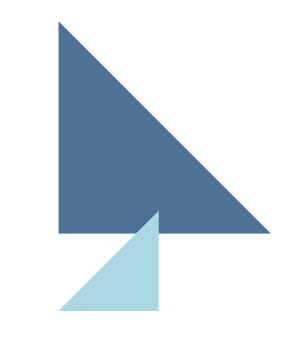
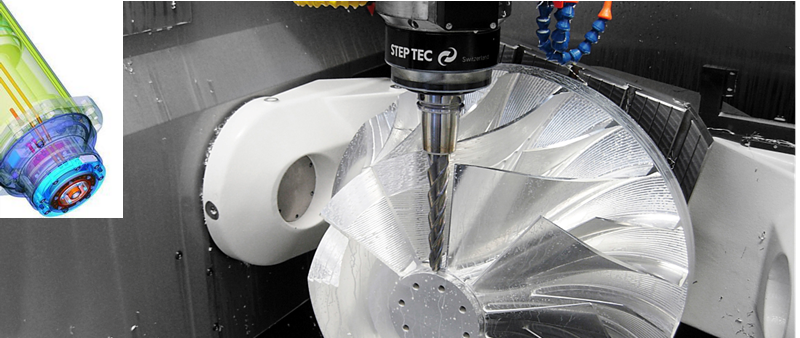
%
Planning time svings
%
Increase of Overall Labour Effectiveness
%
Rejected Spindles reduction
%
Rejected Machines Reduction
Data-Driven Digital Process Challenges
Current manufacturing processes for Spindle production are only partially digitalized, creating significant data gaps across the workflow. This makes difficult the optimization of quality and productivity for the final control and use phases, as teams in charge have lost visibility on critical features in the chain, and there is no global model nor database covering it efficiently.
Big Data Business Process Value
The GF Zero defect factory twin 4.0 trial considers two main business processes for implementation:
Spindle manufacturing: This process involves manufacturing of components by external partners and by internal capacities. The internal process include the design, planning, machining and measurement of critical dimensions of components, the assembly and automated tests for quality control before delivery to the machine manufacturing factory.
Machine Spindle assembly and sales transfer: This process follow up the previous after reception of spindles, through specific assembly and functional tests, requiring access to data from spindle manufacturing stages, and including part manufacturing tests which reveal differentiated aspects of spindle quality performance, in terms of accuracy, precision and productivity, before delivery to sales and customers.
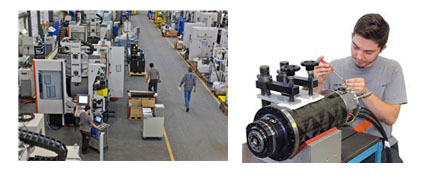
Large Scale Trial Performance Results
The GF trial has provided both a structured approach and an appropriate infrastructure for data collection and aggregation in semantic databases allowing full traceability of the Spindle manufacturing process, and a complete analysis tool of the performance of assembled components during quality tests. Additionally, the trial provides a dedicated dashboard displaying in real time the factory KPIs, and machine learning algorithms delivering predictive quality outcomes as well as critical features correlated with defect rates so they can be anticipated and KPIs improved.
These new capabilities provide transparency to the full manufacturing process. Operators, Managers and Quality teams are now able to follow up the dynamics of the quality indicators, defect rates, production delays, quality iterations, and have access to relevant data, from component references to detailed characteristics, in order to adapt the process smoothly. Quality prediction algorithms are able to identify subsets of spindles going to final tests having high probability of failure, with accuracy of predictions around 95%.
Observations & Lessons Learned
Benefits of full digitalization become significant for a complex Spindle manufacturing process chain, as the accuracy and robustness requirements grow with new applications, which are being developed in markets like aerospace and medical, involving complex geometries and very hard materials.
Improvements of manufacturing KPIs are possible now thanks to detailed root cause analysis drawing from component manufacturing and assembly stages, supporting expert view and showing that design and processes can be still tuned taking into account new findings at functional levels along the product lifecycle. Several events have been for instance identified at machine control level which are related to spindle manufacturing stages and specific components, now reconsidered in the perspective of the Spindle Digital Twin paradigm.
Replication Potential
The potential for replication is sustained by the ontologies which are the base for building the Digital Twin and data model for the Spindle lifecycle. The semantic framework guarantees replicability and scalability to different types of spindles which can integrate a unique database, enhancing the robustness of predictive analytics and machine learning models, which can be deployed now to machine levels and usage phases of the product.
Milling and Spindle Factory
GF Machining Solutions
Biel | Switzerland
Pilot Partners
Standards used
- OPC UA
Big Data Platforms & Tools
- MS Azure
- Python
Big Data Characterization
Data volume
2TB
Data velocity
1GB/day
Data types
- CSV-JSON
- System Logs
- CAD-CAM
- Statistical data
- Sensor/machine data
Number of sources
- Environmental param.
- Machine configuration
- Machine conditioning
- Operational data
- 3D models
- Quality systems
- CMM data
Open data
No
Implementation Assessment
Technical feasibility
“Successful and exemplary implementation of big data and analytics in a GF factory with the latest SW tools and technologies”
Economic feasibility
“Business potential is significant at levels for factory efficiency, with clear cost saving benefits, and for market share improvement with the quality increase of the products”
Replication potential
“The pilot is designed to be highly replicable and scalable thanks to developed ontology and semantics taking into account future deployment accros GF factories and beyond”.