ZDMLytics
ZDM for flexible manufacturing based on Data analytics-driven Variation Detection and Analysis
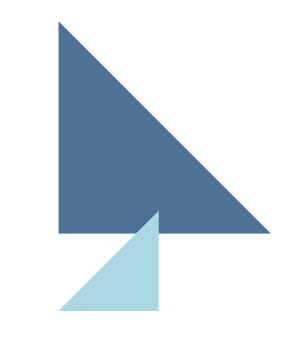
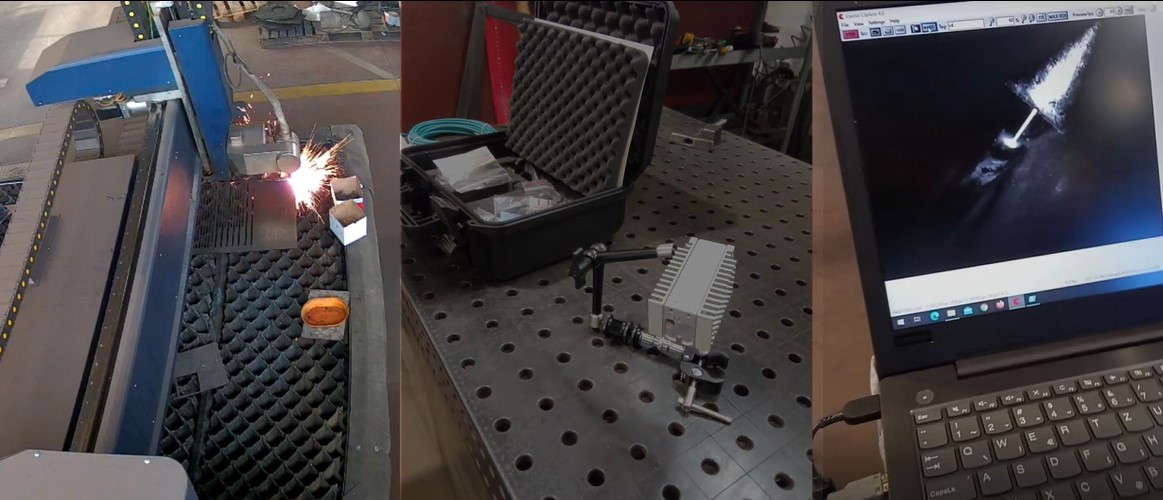
Nissatech is an innovation-driven SME with strong international cooperation and vision to become one of European top innovators in the domain of advanced AI and cognitive industrial solutions. The main objective of the company itself is to develop own technological building blocks through an efficient implementation of the cutting-edge research and their usage for resolving very challenging real-world problems in different industrial domains.
Data-Driven Digital Process Challenges
The “tool change” is the activity where the tool on a machine should be changed due to its degradation over time (usually after several thousand of usages, which can be even daily for a high-production capacity. It is usually implemented as a planned tool maintenance task, where the total usage of the tool before being replaced is predefined. The plan is based on the recommendations of the tool vendor as well as on the workers experience. However, this is not the most efficient approach, since it doesn’t exploit the individual characteristics of the usage of a concrete tool. Indeed, it is well known that the tool degradation depends on many factors specific for a concrete tool, like the concrete production process that tool is applied in, the quality of the supplied material to be processed by that tool, to name but a few.
From the system behaviour theory point of view the tool wear belongs to the class of issues which cannot be resolved easily since 1) the numerical models for tool wearing are not existing (except in some special cases) and 2) the data from the past tool degradation cases is usually useless for learning how the tool degrades since the tools are changed in a predefined way, well before the tool would be broken (what would produce very useful data). More precisely, tool wear belongs to unpredicted undesirable situation, the case when no data and no models are available for understanding the system behaviour.
Big Data Business Process Value
ZDMlytics treats tool wear as a problem of predicting, as precise as possible, when the unusual-anomalous behaviour of a concrete tool, caused by the wear/degradation process, will lead to the problem in the quality of the product. However, this prediction cannot be done using traditional data analytics approaches, since the data describing this transitional behaviour is missing. On the other hand, usually there are huge datasets available from the past manufacturing processes, which could be used for supporting above mentioned prediction process.
ZDMlytics combines the precise prediction of future trends in process data (relevant for the tool degradation process) and robust detection of anomalous behaviour in such trends, indicating that the tool has degraded significantly and should be replaced.
Large Scale Trial Performance Results
The development consists on big data driven (predictions) and a later that is model based (multivariate quality control – variation detection). This combination of a) predicting data that is missing which corresponds to simulating the behaviour of the tool as it would be not replaced as planned and, b) observing variations/instabilities in such a behaviour which corresponds to understanding the effects of the degradation process, compensate a huge uncertainty inherent in this process.
Value chain
Observations & Lessons Learned
This approach can be very useful for an efficient and affordable anomaly detection in a wide set of cases related to IIoT.
The main advantage of this solution is that the taken approach offers a scalable data-driven development of complex models in order to enable real-time multi-parameter monitoring of different processes.
This new development will enable a transformation of manufacturing systems from reactive into proactive. That is, the production systems will be able not only react on a problem at hand, but rather to sense the problem in advance and proactively resolve the situation/anomaly (ahead of time).
Replication Potential
Replication potential is huge. This project probes that ZDMlytics can be applied in different tool wear scenarios since it is a data-driven approach and it does not require a huge involvement of human experts (for modelling). In addition, the data-driven model can be created from past data using advanced ML methods.
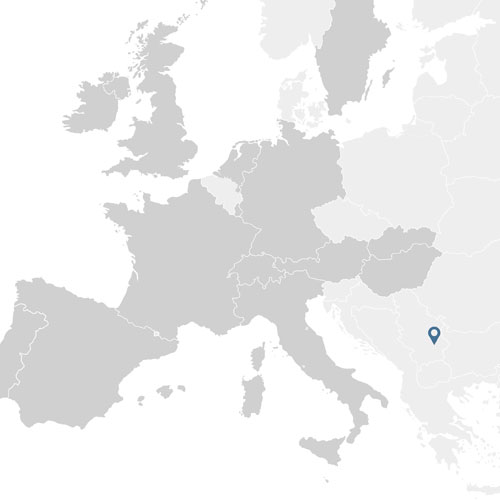
EMDIP, Serbia
Pilot Partners
Digital Platforms & Tools
- D2Lab
- QARMA Enabler
Big Data Characterization
Data Volume
20 GB
Data Velocity
0.03GB/day
Data types
- Measurements
- Statistical data
Number of sources
- 4 powers
- one temperature
Open data
No
Key Facts & KPIs
Productivity
PR1 – Production cost reduction 30%
Sustainability
SU1 –Waste and material reduction 30%
Agility
AG1 – Visual inspection reduction 80%
Adoption Assessment
Technical feasibility
Economic feasibility
Replication potential