Virtual Massive Metrology
Trimek Trial: Zero Defect Manufacturing Powered by Massive Metrology
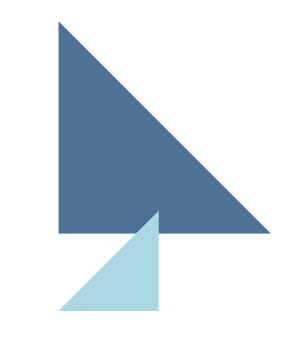
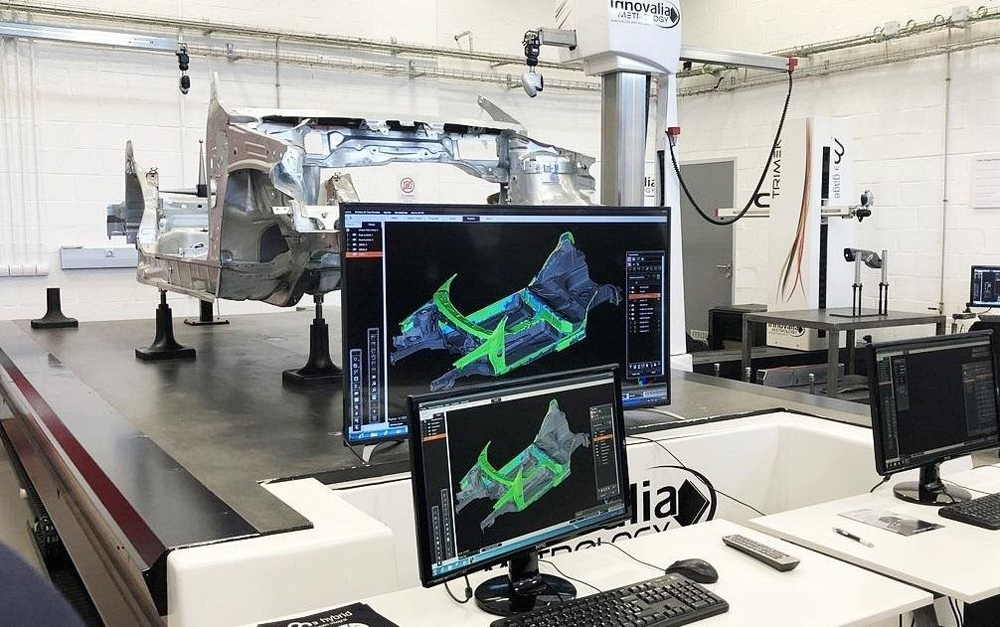
%
measurement planning and programming times reduction
%
massive point clouds acquisition & processing time
%
products rejection reduction
%
cost reduction through faster decision making
Data-Driven Digital Process Challenges
Precision dimensional quality control is increasingly present in the manufacturing industry, and measuring requirements are increasingly demanding. The imperative need to optimize the assembly processes entails the requirement for greater precision in the production of components and parts to be assembled, and therefore, quality control is more demanding.
Big Data Business Process Value
The Trimek ZDM Massive Metrology 4.0 trial considers two main business processes for implementation:
High-density metrology: This process has developed a system capable of rapid acquiring and processing big volume of 3D point cloud data from complex parts and analyzing massive point clouds coming from those parts by means of advanced 3D computa-tional metrology algorithms, obtaining a high-performance visualization, that is, a realistic 3D colour map with textures.
Virtual Massive Metrology: This business process has developed a digital me-trology workflow and agile management of heterogeneous products and mas-sive quality data, covering the whole product lifecycle management process and demonstrating an advanced QIF metrology workflow, starting from the product design process up to the advanced analysis and visualization of the quality information for decision support.
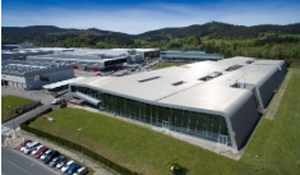
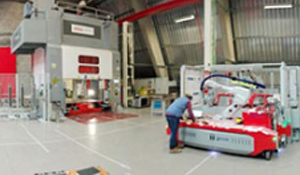
Large Scale Trial Performance Results
The Trimek trial has allowed the implementation of advanced metrology 4.0 algorithms based on 3D computational capabilities to finally obtain a texturized mesh, instead of an annotated polygonal mesh, that is a more realistic visualization of the physical product for human a centered decision support process. Large pieces have been scanned and analyzed and the trial has demonstrated that the BOOST 4.0 big data pipelines are capable to process the whole body of a car.
These new big data capabilities have allowed the automotive industry to work fluently with 10 times larger CAD files, which is important as having all the car modelized. The processing speed has also been multiplied by 5, which allow a bet-ter performance and a more fluent analysis and visualization. Also, the time needed to program the scan of each piece has been reduced significantly, up to the 80%, as having the whole car already modelized allows for a digital planifica-tion of the trajectories thus reducing the time needed to configure the scan.
Observations & Lessons Learned
Bringing our data in one semantic model provide cost saving value in a short term and in the later steps of analysis. Boost 4.0 trialed and discovered also new ways and new standards to bring data together and set up a manufacturing Digi-tal Twin, highlighting the benefits internally during the project.
As a result, some critical improvements are now possible: machine learning al-gorithms help to achieve unexpected accuracy (even with limited data) by detecting manufacturing issues; semantic and QIF standards give the possibility to link data together in an efficient way, and the cloud solu-tion gives the possibility to deploy easily AI in testing environments and integrate them through APIs in any platform, making the full deployment swift and valuable.
Replication Potential
As a result, some critical improvements are now possible: machine learning al-gorithms help to achieve unexpected accuracy (even with limited data) by detecting manufacturing issues; semantic and QIF standards give the possibility to link data together in an efficient way, and the cloud solu-tion gives the possibility to deploy easily AI in testing environments and integrate them through APIs in any platform, making the full deployment swift and valuable.
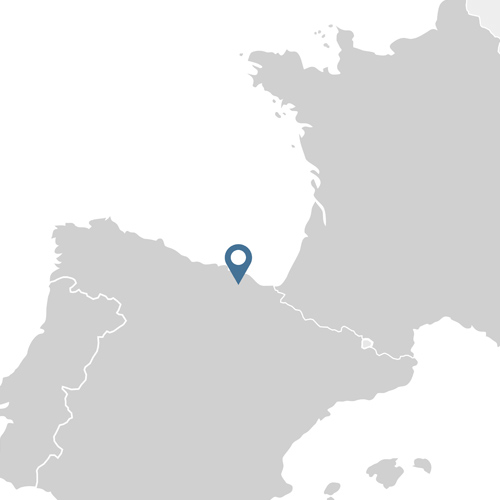
Automotive Smart Factory, Automotive Intelligence center (AIC) Boroa | Basque Country, Spain
Pilot Partners
Standards used
- QIF
Big Data Platforms & Tools
- M3 Cloud
- Redborder
Big Data Characterization
Data Volume
4TB
Data Velocity
150GB/day
Data types
-
Pointclouds
-
GD&T
-
Colourmapping
-
Statistical data
-
MBD
Number of sources
- Environmental params.,
- Machine configuration
- Machine conditioning
- Operational data
- Cad model
- 3D Scanner
- CMM condition
Open data
No
Implementation Assessment
Technical feasibility
Economic feasibility
Replication potential