Unsupervised Anomaly Detection System TNO
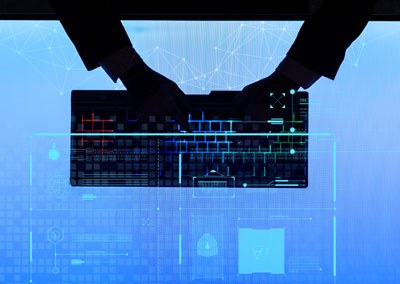
Do you recognize the problem of generating a lot of (visual) data and the challenge of interpretating these data in order to understand your production operation, let alone controlling the products coming out of your production line? Very often these datasets are overwhelming and can not be analysed on-line and on time. For this reason TNO developed a new unsupervised algorithm for a specific dataset. It was tested with real production data. It is expected that this type of algorithm can be implemented in many different situations to allow production to be up and running with good quality data analysis in a short time.
The enabler consists of Deep Learning network that can be applied on images of a fixed format. The Deep Learning network maps these images via a number of (convolutional) layers to a latent space of a fixed dimension. Next the decoder in the network reconstructs an image in a reverse version. By training the network on a set of images the network learns to reconstruct images constituting the bulk of the dataset. The reconstruction error can be used as a measure of the deviation between the actual and the desired product. When the reconstruction error exceeds a certain threshold value the product is considered as anomalous
The algorithm was tested off-line on a dataset of a high volume product from one of the pilot line factories in Qu4lity. It was concluded that it catches twice as many true defects (True Positive Rate is almost doubled), whilst maintaining the low number of false rejections
The algorithm is soon to be further tested in the actual production environment.
For further information please contact us at: flexman@tno.nl