Smart digital engineering process using industrial big data – closing the loop validated knowledge engineering
Fill Pilot
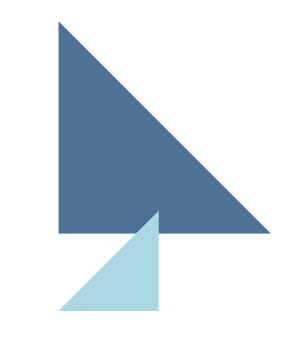
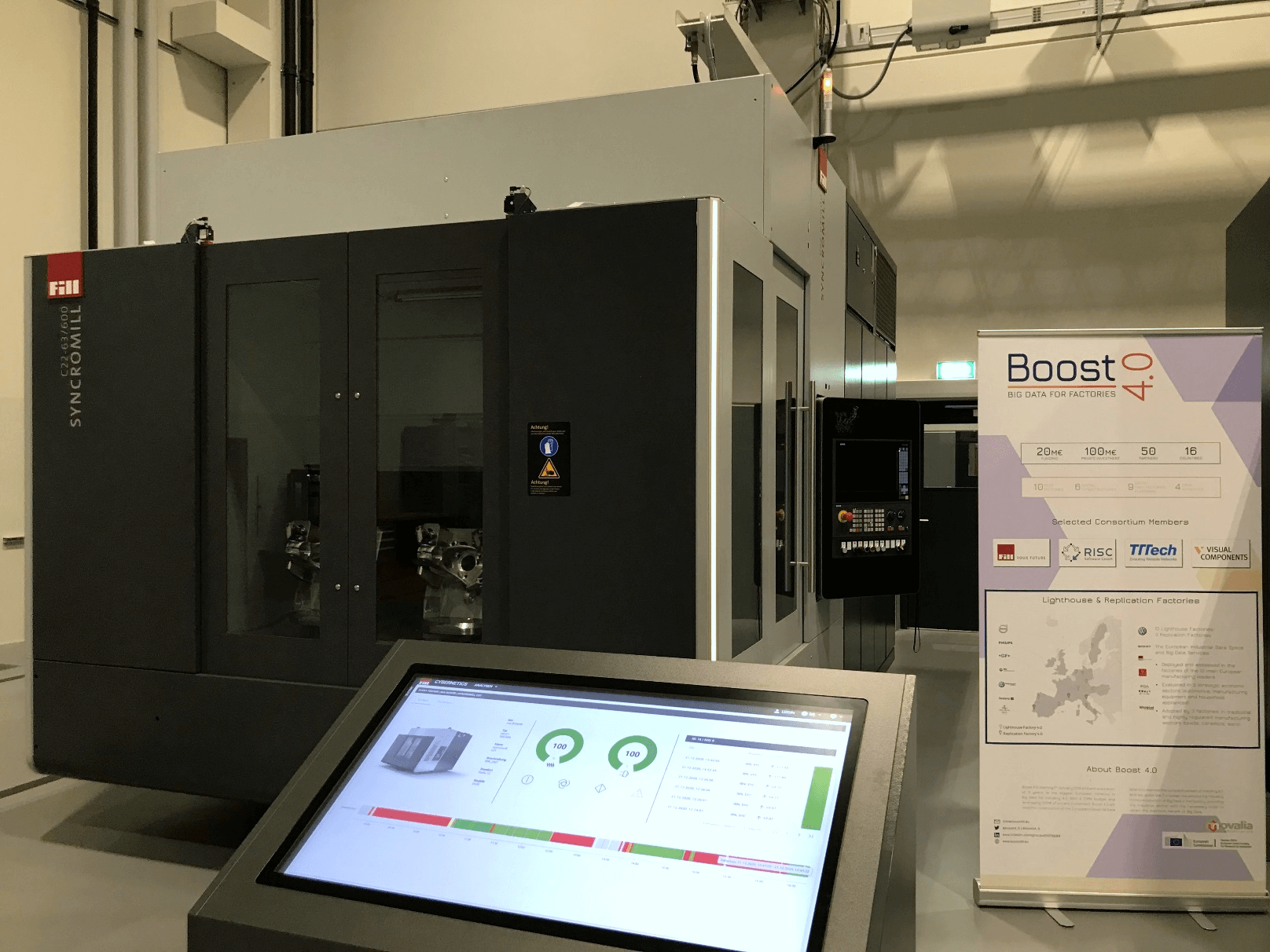
%
Reduction of engineering lead time
%
Reduction of failures due to design faults
%
Reduction in service costs
%
Reduction in commissioning time
Data-Driven Digital Process Challenges
The expansion of competitiveness and sustainability are fundamental goals of companies. To achieve this, every business needs to deploy digital technologies in a variety of areas, including customer relationships and services, productivity, business model, IT security, and privacy. Digitization and networking are playing an increasingly important role, as the digital data volume will increase significantly.
The growth speed of the data volume, the diversity of the data and the various data sources pose many challenges, such as a collection of sensor data and the mapping of model data underlying the machine, their integration and interpretation into a structured database system.
Big Data Business Process Value
Within the CAx systems, the optimization of products, e.g. machine tools, are done by engineer’s expertise and their empirical knowledge. Several loops are performed to optimize products and production processes to fit the customer needs. Thus, customization is a lot of work and time-consuming. During the sales and project-planning phase in many cases, no simulations of the process or valid process data are available for frontloading to minimize the risk for the machine builder.
Implementation of Big Data Solution
The V-model (compare VDI 2206) describes the development of mechatronic systems based on a systematic analysis of the requirements and a distribution of the requirements and loads among the individual disciplines. The detailed development then takes place in parallel and independently of each other in the individual disciplines. The results are then integrated into subsystems and systems and validated regarding compliance with the requirements.
The new proposal for an integrated business process for smart digital engineering using big data extends the V-model by (Figure on the right)
- Agile model management & development process (model repository)
- Data analytics process (involving data analysis and machine learning methods)
- Service development process
- Simulation based release process for product service systems
Large Scale Trial Performance Results
To give insights on the performance, the following objectives have been defined and measured in the engineering process on Fill products, accurate robotic NDT systems accubot® and machine tools syncromill ®.
Long-term reduction of machine development times (estimated -15%, achieved -26,4%) or the option for better, more innovative concepts.
Unplanned downtimes (estimated -20%, achieved –20,8%)
Service cost reduction (estimated –15%, achieved –19%)
Simulation based release process reducing commissioning time (estimated –10%, achieved –14,7%)
Observations & Lessons Learned
The development of innovative, highly customized production systems is generally based on a customer-centric approach. Furthermore, for the machine builder, the operations process knowledge is a key success factor. This is mostly expertise and empirical knowledge and is usually built up during the operating phase and used in follow-up projects. The intended feedback loop should accelerate the build-up of knowledge and make it possible to secure customer needs and even wishes earlier and increases customer satisfaction. This leads to fewer delays in the business process like the customer dependent approval processes, e.g. design approval, delivery approval, etc. This leads in the following to earlier cash flows which are linked via a payment schedule to the approval process
Fill GmbH
Gurten | Austria
Pilot Partners
The extended V-model shows the integration of all four business processes.
Alois Wiesinger, CTO Fill
“Fill is a grown family-owned company and focused on its customers success. The new approach of closing the information gap by digitalization between customers and the Fill engineering leads to a better fulfilment of customer requirements. By end of Boost 4.0 we already notice a higher level of customer satisfaction. In addition, the high availability of industrial data across companies enables digital, agile and self-controlled production and value added networks. Besides the facts and figures of the measured KPIs, it is about the working people and their motivation. I think the digitalization and its approach is mandatory, but to benefit from it, it is about the mindset to see digitalization as a chance and not a threat. With this newly created transparency on the process and the trust we have built up with our partners and customers we are best prepared for the factory of the future!”.