Philips pilot enters testing phase with TTTech Industrial
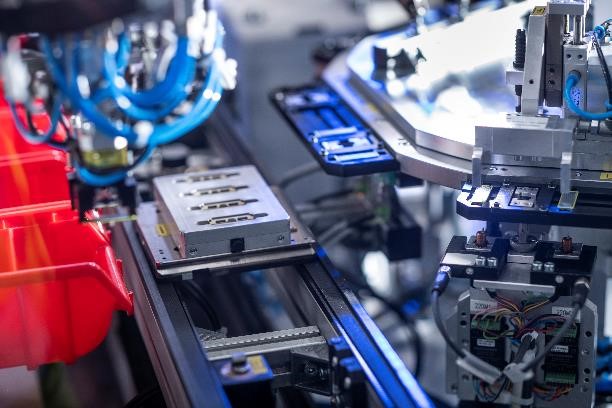
A pilot led by Philips, with contributions from TTTech Industrial, TNO and Fraunhofer IPA, is currently being tested.
Within the QU4LITY project, Philips is working on methods to improve and enhance its quality control for the OneBlade production lines in cooperation with partners from the Qu4lity consortium. For one quality control task, Philips and TNO are exploring a new method in the form of unsupervised learning for the quality control of the pad-print which is applied on the cutter. TNO has developed an algorithm that can be applied in the pilot and tested this algorithm based on an initial dataset with very promising results, increasing the accuracy of the quality control resulting in catching more defects while maintaining a low percentage of false positives. The next steps are to stream quality control images to the edge computing solution of TTTech Industrial in order to run the algorithm on live data. In addition, Philips is working with Fraunhofer IPA to develop a method to predict torque values of the product based on data from preceding production steps. By doing this, both partners hope to catch defects earlier and increase the productive capacity of the production line. Currently IPA has made advancements in the development of the algorithm and in order to validate this, Philips is collecting an enlarged data set after which they are looking to employ the edge computing solution of TTTech Industrial to deploy this algorithm on live data.
Nerve, the edge computing solution that TTTech Industrial is bringing to the Philips pilot, acts as a backbone capable of communicating the required data for zero defect manufacturing (ZDM). In close cooperation with the other partners, TTTech Industrial supports the integration of the edge computing technology into the existing pilot line. TTTech Industrial has further developed its open edge computing platform Nerve as a modular system for factory automation by enhancing various functions such as PLC control, for applications like condition monitoring, anomaly, or threat detection.
As mentioned above, TNO developed a new unsupervised algorithm for a specific dataset of the Philips production line. The enabler consists of a Deep Learning network that can be applied to images of a fixed format. The Deep Learning network maps these images via a number of (convolutional) layers to a latent space of a fixed dimension. Next, the decoder in the network reconstructs an image in a reverse version. By training the network on a set of images, the network learns to reconstruct images constituting the bulk of the dataset. The reconstruction error can be used as a measure of the deviation between the actual and the desired product. When the reconstruction error exceeds a certain threshold value, the product is considered as anomalous
The algorithm was first tested off-line on a dataset of the Philips pilot line factories in Qu4lity. It was concluded that it catches twice as many true defects (True Positive Rate is almost doubled), whilst maintaining a low number of false rejections. The algorithm is soon to be further tested on live data in the actual production environment. Fraunhofer IPA develops an ML based system that detects anomalies in the torque of the final product. It considers data from preceding production steps. This includes the station where the moving parts of the shaving elements are assembled. In the end, the objective of the system is to identify process anomalies as early as possible in the process chain and to enable corrective actions.
The key input to the anomaly detection are high-frequency time series from sensors that are integrated in the production line. In the course of the project, correlations have been identified with force-way-data (from the key slide-in movement) and with encoder data from involved robots that perform placements of the components. Different approaches have been developed to extract meaningful information from the raw sensor data. Good prediction results have been reached with support vector classifiers. Classifiers based on neural network approaches have been considered as well.
Anomaly detection was first tested with off-line data from a Philips pilot line within Qu4lity. In a next step, the ML process will be implemented on an edge device provided by TTTech Industrial that is located at the production site. The anomaly detection software on the edge device performs the ML based inference (i.e. the evaluation of the current/previous assembly cycle) in combination with other auxiliary tasks like data pre-processing, data fusion or database storage. Complex tasks like the training of the ML model can be performed locally as well or alternatively in an external cloud.