OTIS Factory 4.0 elevator production
OTIS: Zero Defect Manufacturing Powered by Data analytics and E-IDS/FIWARE secure & flexible data sharing
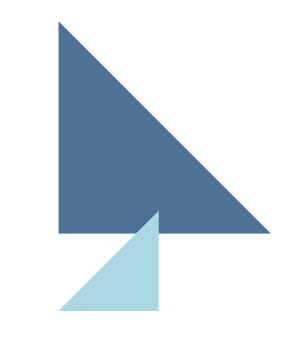
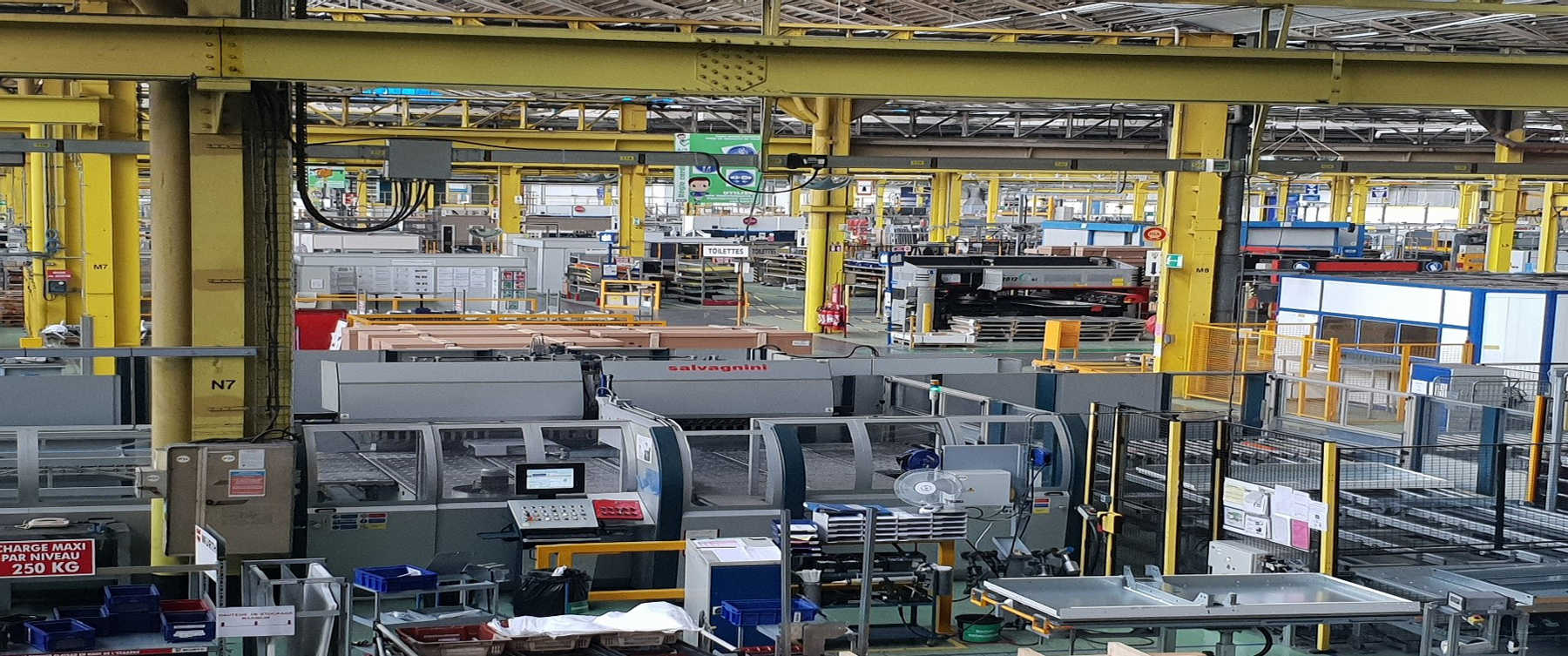
%
Additional Material Transfer (AMT) reduction
%
Overall Equipment Effectiveness (OEE) improvement
%
Increase production line machines availability
%
Improve process (doors and panels) quality
Data-Driven Digital Process Challenges
Data insights acquired during panel and door production process helps OTIS reduce scrap material events, streamline production and optimize machine usage. Integration of different big data silos using BOOST 4.0 technology enabled creation of new optimization decisions in the production process.
Big Data Business Process Value
OTIS replication pilot focus on detection and prevention of the faulty operations and machine-breakdowns by using two main processes:
Data Silos Integration and Data Analytics: Integration of different manufacturing data sources to gain advantage and optimize production line processes was main focus of this process. Analysis of cleaned machine and MES systems data provides insights to help improve OEE and reduce TPM2 error in the panel production line.
Secure Supply Chain Data Sharing: AMT reduction is achieved by enabling production floor to securely exchange part of production data with suppliers and business partners. Optimization achieved is obtained through using E-IDS/FIWARE connector/broker integrated to manufacturing process enabling suppliers and partners deliver material on time and provide near real time feedback about the product installation bringing OTIS factory closer to Factory 4.0 standard.
Large Scale Trial Performance Results
OTIS panel production line simulation results with optimized production model showed significant savings over before change production line. The new approach helps detect unscheduled machine and line faults and anticipates manufacturing line problems ahead of time. The outcome yields 10% in AMT reduction and OEE improvement as well as 12% machine availability and 5% on production quality. AMT and OEE results are influenced by machine on the line monitoring that enhances chances of capturing problems before they create larger problem thus increasing OEE as well product quality as machines is serviced on time. The difference between KPIs and machine availability comes from the fact of time lost between shifts and personnel change. The quality improvement comes from properly serviced machines that don’t require much intervention during production process and use less material to produce desired panels.
Data sharing through E-IDS/FIWARE connector enables OTIS supply chain and partners to further optimize production by reducing line stops due to lack of material and optimizing book of orders in case if product is required on the field as replacement.
Observations & Lessons Learned
Production data understanding is critical in model creation. The comprehension fidelity comes from knowing all aspects of the production process and looking into lots of detail. Another point to note especially when looking at data correlations is data engineering (cleaning, wrangling, alignment etc.). This step is in many cases omitted resulting in incorrect model designs and might result in missing optimization opportunities.
From connector and data/model wrapper implementation and integration using state of the art technologies (microservice architecture – containers) to integrate model into BOOST 4.0 ecosystem was significant as it simplified deployment and integration work.
Replication Potential
Realized deployment proved technical feasibility of replication developed technologies have been tested successfully on FIWARE (Germany) and SQS (Spain) premises. Rancher and Docker container technology are used to simplify replication of the components to new production environment. BOOST 4.0 technologies HW footprint minimalization increases replication potential. This work is done by FIWARE to enable deployment on small form factor industrial computers. The economic feasibility depending on the scale of replication work will vary (HW/SW and experts time). From technologies point of view the replication potential is medium and will depend on the scale of new deployment and type of the processes that require Factory 4.0 transition.
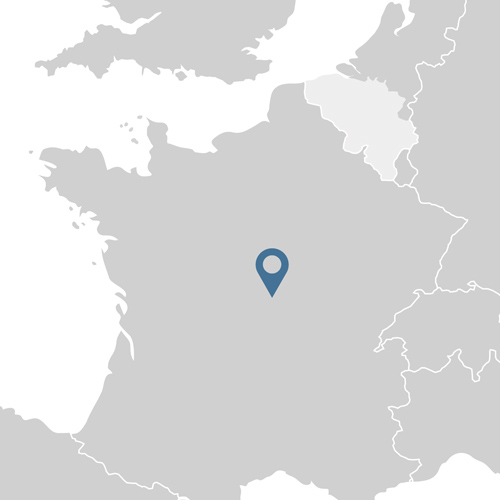
Smart Factory, OTIS Gien production facility, Gien, France
Pilot Partners
Standards used
- Plataform INDUSTRIE 4.0
- FIWARE NGSI
Big Data Platforms & Tools
- MosQuitto
- Fiware
- Rancher
- Anylogic
- Power BI
Big Data Characterization
Data Volume
~200GB
Data Velocity
500MB/day
Data types
- Machine operations
- MES
- ERP
Number of sources
- Machine data
- Machine configuration
- Machine faults
- Operational data
- Book of orders (ERP)
- MES – order in production
Open data
No
Implementation Assessment
Technical feasibility
Use cloud and microservice architecture provides replication flexibility to many Factory 4.0 scenarios.
Economic feasibility
Deployment cost depend on the scale of the deployment and can be tailored to required solution budget.
Replication potential
Replicability potential will vary depending on the new problem type and scale of the environment.