MONDRAGON CORPORATION – FAGOR ARRASATE – DANOBAT GROUP
Zero defect and autonomous quality in machinery building for capital goods sector
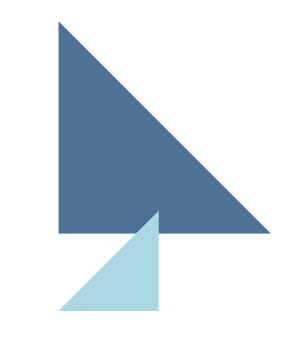
MONDRAGON CORPORATION is a global technology business group, a cooperative alliance shaped by mutually independent cooperatives that bases its growth in the excellence, innovation, quality, social commitment and internationality. Being the biggest industrial cooperative group world-wide active in more than 120 countries, it operates on the areas of manufacturing industry, finances and retail
FAGOR ARRASATE is a Company of 800 employees specialized in design, manufacturing and supplying sheet metal forming machine tools. FAGOR ARRASATE was created in 1957 and, since then, has expanded its products portfolio and business in an extraordinary significant manner, being now one of the world leaders in the field. It is one of the 5 biggest manufacturers in terms of turnover and the first one considering the products portfolio.
Founded in 1954, DANOBAT is part of the DANOBATGROUP which is one of the largest machine tool and production system manufacturers in Europe (that has over 50 years’ experience in supplying high precision equipment and innovative solutions to many industry sectors).
Process Challenges
MONDRAGON proposes two process pilots in the Machinery Building for Capital Goods scenario; two realities that can be complementary in many customers’ value chains:
1) Manufacturing Processes with Cutting/Grinding Machinery, led by Danobat
2) Manufacturing Processes with Hot Stamping Machinery, led by Fagor Arrasate
Each manufacturing process has it’s own distinctive features but a single goal: to go a step forward ZDM process towards AQ in manufacturing processes and products. Challenges are related to IIOT platform, Data Analitics of the variability of the process, interoperability and standardization considering the heterogeneous devices and data involved. All of them with the objective to address ZDM and Autonomous Quality. Main challenges in the Mondragon pilot relate to:
- Lack of horizontal integration makes the identification of cause effect relations in heterogeneous systems even harder, becoming the different steps of a productive process in information silos where there is not any information exchange in order to identify inter process issues.
- Several Deviations/Anomalies or process variability. Several analyses should be processed for providing action points in the improvement of the manufacturing processes with the support of AI algorithms.
- Lack of Standardization. Manufacturing Processes should be carried out having into account standards in communication, data format, systems and devices representation in the manufacturing value chain
Process Value
The production lines of Danobat have selected as the most representative multi stage zero defect manufacturing pilot demonstration for the QU4LITY platform. The integration of sensors , PLC, CNC and IIoT platforms will provide a ZDM approach that will impact the production of the lines provided by Danobat.
The automotive world is a sector that is in constant movement and where technological developments require a continuous technological renovation The development of this project will allow FAGOR ARRASATE to offer to its customers a more accuracy, competitive and productive hot stamping lines and a greater degree of sophistication with respect to what the market currently offers. The development of this project will facilitate the increase of the level of sales.
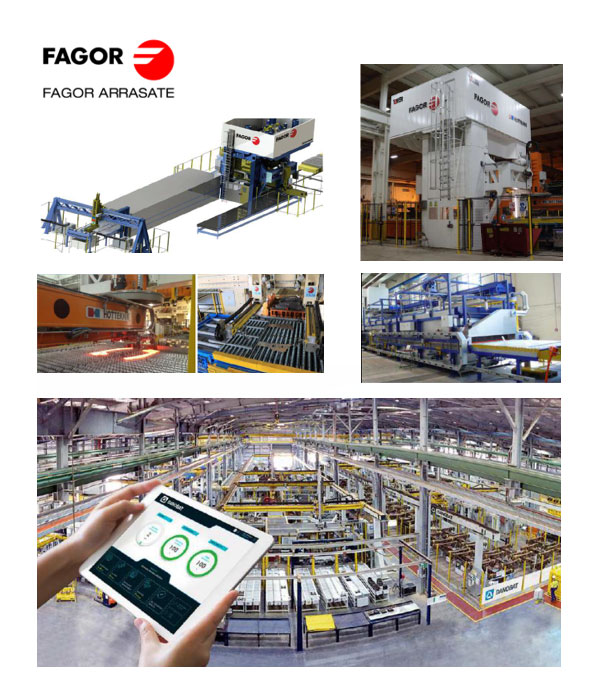
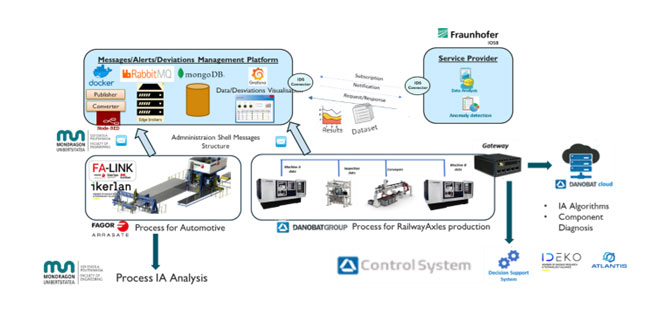
Experiment Performance
- Component Diagnosis and Anomaly Detection ( fingerprint and pattern recognition for RUL calculation for DANOBAT)
- Component Diagnosys and Acustic Anomaly Detection for DANOBAT
- Machine+ Checking Station for faulty parts identification
- Fault Detection: LSTM and CPD algorithms. Matrix Profile Algorithm
- Fault Classification: MASS, DTW , MPDist
- Control system by IDEKO prioritizes the schedule and work orders
- IKCloud+: Machine Learning Operations (MLOps) solution that provides end-to-end workflows for model development and deployment for FAGOR ARRASTE Scenario
- ikCloud+ has been plugged into FA-Link, providing support for ML model-based data analytics for FAGOR ARRASATE Scenario
- Converter for Interoperability Enabler by MGEP enables syntactic interoperability between heterogeneous systems as a proof of concept for translation of data formats The solution has been demonstrated
- Messages System Management: Data access and ingestion through the Edge Broker. The Edge Broker is composed of Publish-Subscribe servers.
- Data Exchange connectors to enable data Gobernance
Value chain
Observations & Lessons learnt
- ZDM and AQ apporach has been defined and deployed
- Integration of sensors, PLCs, IIOT platforms, Digital Converters , Industrial protocols, Edge-Cloud Layers,… have been key for addressing digital challenges. Hybrid and flexible Edge-Cloud Architecture is necessary
- DevOPS and OpenSource are key from technological and business perspective
- Standardization helps to structure data, interoperability and semantic knowledge
- It is required pre-processed and ready data and key variables for Data Analytics. Multivariate, Semi supervised Anomaly Detection, MLOPS, Long short-term memory in Deep Learning ,RUL have been some of IA strategys carried out Manufacturing Processes have boosted early detection of anomalies, OEE, machine downtime and process execution and scheduling
- First implementation of Manufacturing Data Spaces with IDS connectors
Replication Potential
- Architecture of the solution could be applicable in other implementations, specifically for MLOPS and anomaly detection.
- Standardization considering Asset Administration shell and OPC-UA and IDS help to structure data and messages to implement in future Edge-Cloud/ IT-OT solutions cross factories for sharing data
- Strategy for Interoperability between heterogenous devices is replicable, although with highly customization needed
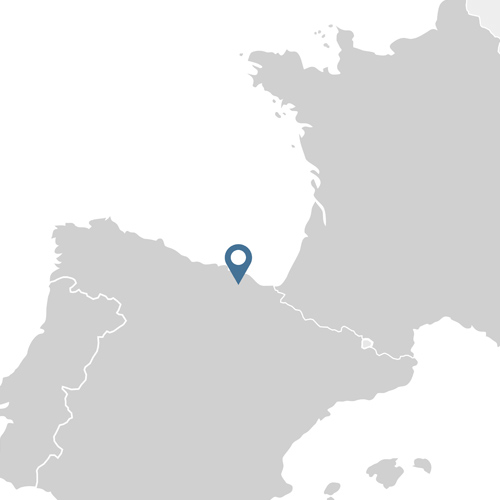
MONDRAGON CORPORATION – FAGOR ARRASATE – DANOBAT GROUP, Spain
Pilot Partners
Standards used
- IEC 62541-5:2020 OPC Unified Architecture
- IEC 63278-1 ED1 Asset Administration Shell
- ISO/IEC 21778:2017 – JSON data interchange.
- DIN SPEC 27070:2020-03 Requirements and reference architecture of a security gateway for the exchange of industry data and services
Digital Platforms & Tools
- SAVVY IOT
- FA-LINK
- IKCLOUD + IKSEC- MLOPS
- Digital Converter+ Messages System Management
- Atlantis Decision Support System
- IDS data exchange connectors
Big Data Characterization
Data types
- Griding Machine data [seconds, Speed, Load, Temperature]
- Press Machine Data [ ºF, kN,s]
Number of sources
- Griding Machine data (CNC controller, Linear Axis , Rotary Axis)
- Press Machine Data (Oil temperature, Pressure central cilinder, Furnace real time, Water flow input..)
Open data
No
Key Facts & KPIs
Productivity
PR1 – (ex. Factory output increase)
PR2 – (ex. Productivity increase)
PR2 – (ex. Product cost reduction)
Speed
SP1 – (ex. Speed to market reduction)
SP2 – (ex. Design time reduction)
Sustainability
SU1 – (ex. Waste and material reduction)
SU1 – (ex. Energy efficiency)
Agility
AG1 – (ex. Lead time reduction)
Customization
CU1 – (ex. Configuration Accuracy)
Adoption Assessment
Technical feasibility
Economic feasibility
Replication potential