Big Data Value Spaces for Competitiveness of European Connected Smart Factories 4.0
PCL Pilot: Autonomous short-batch injection moulding production process
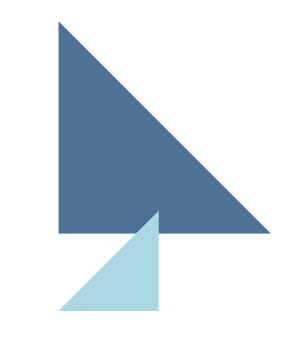
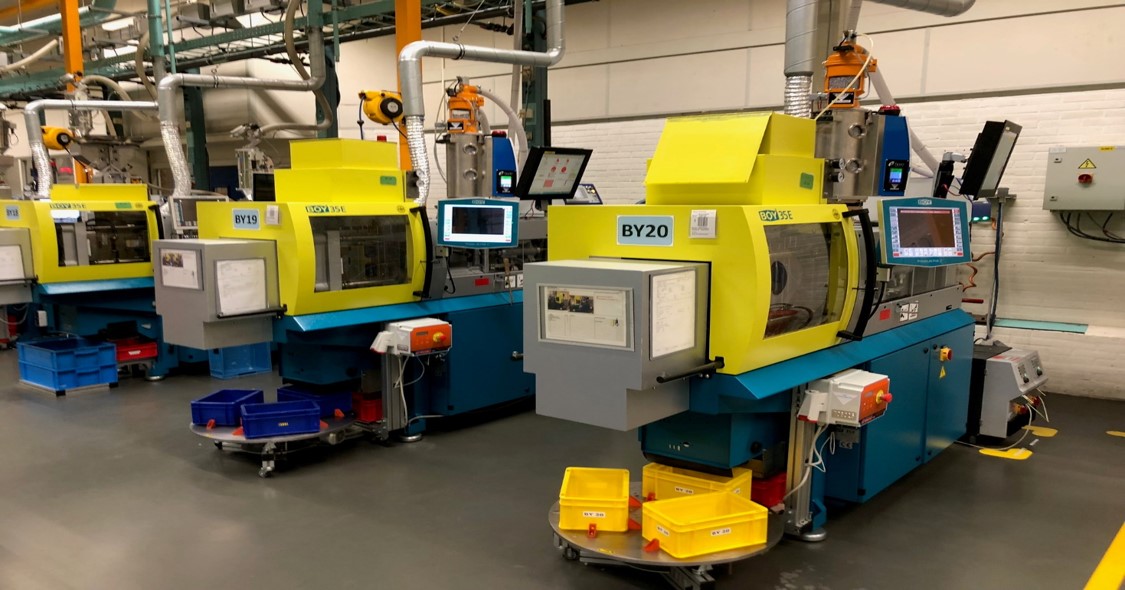
%
Predictive Quality - Improvement on Fall Off Rate
%
Predictive Maintenance – Less downtime
%
Mean Time to Repair improvement
Standard approach for connecting IM processes
Data-Driven Digital Process Challenges
Plastic injection moulding is a competitive market and it is essential for PCL to continuously improve on quality, production performance, and costs where this process is concerned. The application of Big Data technologies and fact-based decision making, along with seamless connectivity in the manufacturing process, will result in more efficient ramp-up times between different moulds, higher production output, better quality and with full traceability along the process chain all the way to the customer.
Big Data Business Process Value
Standardized approach for connecting IM machines: A solution has been constructed that allows the ‘mapping’ of a generic data stream from the IM machines. PCL uses a pre-defined data model, which map existing data onto this model. IT experts can therefore deploy the data models much faster when connecting new machines. By enabling an automated mapping solution it is possible speed up the scalability towards other IM machines as well as, potentially, for other machines setups.
Model for predictive analysis: The purpose of this model is to be able to make predictive maintenance possible within the PCL plant, scoped to their injection moulding machines. The model is trained and tested with the available (raw/pre-processed) datasets from the moulding machines, providing insights and root-cause analysis towards the operators.
Large Scale Trial Performance Results
It is concluded that the IT-technological perspective for managing the Boost 4.0 platform works well and is also generating value towards its users. Since the start of the pilot, more than one million injection moulding shots have been collected and stored. Each shot contains around 80 parameters, and the data from the six connected machines can be easily shared with other partners.
For the platform to function as expected PCL and IMEC have collaborated on defining a common data format, which IMEC has used to finalize models for understanding the semantics for managing and labeling the data.
Due to the current situation, PCL is not able to embed the technical solutions in a self-sustaining production-mature environment. However, the documentation has been completed and an approach has been agreed on how to train operators in the future. Other personnel, such as line managers, IT engineers and Data Scientists, will also collaborate more closely to support the platform and utilize the operators in using it on daily basis.
Observations & Lessons Learned
The main (technical) elements that are used at PCL are based on open-source tools and can easily be changed / replaced if needed. This allows for a low cost, highly flexible solution that proves to be robust for production environment. It also allows easy and secure exchange of data between pilot partners using the EIDS platform, or any other solution.
A big challenge in Industrial IoT is translation of protocols and the concept of data models. Data models describe the generic lay-out of the data, and IMEC made a sketch of such a model based on PCL input, as well as the Euromap-standards for plastic part making machines. This helps to reduce the time it takes to makes these models significantly, with far less errors, and this improving data quality.
The final steps of the industrialization still has to implemented, however Boost 4.0 is already considered a success within PCL. The concept of data-driven manufacturing, the concept of machine learning and the philosophy of the industrial data spaces has helped promoting Boost 4.0-concept within the Philips global management teams, Philips global IT, PCL local management team, supporting departments as well as operators on what the value of data is.
Replication Potential
PCL has been set up the pilot almost entirely with open source software, which is combined with breaking down our solution into functional clusters. Each cluster is split into functions and deployed as micro-services. These micro-services are highly robust and can be added or removed independently of other functions, making the entire solution extremely flexible and adaptable, which can be used by other partners.
PCL teamed up with replication pilot Ria Stone, which has been beneficial for both partners: PCL has been able to confirm that its solution seems to be a preferred choice and Ria Stone have been able to avoid the pitfall PCL has encountered throughout the pilot.
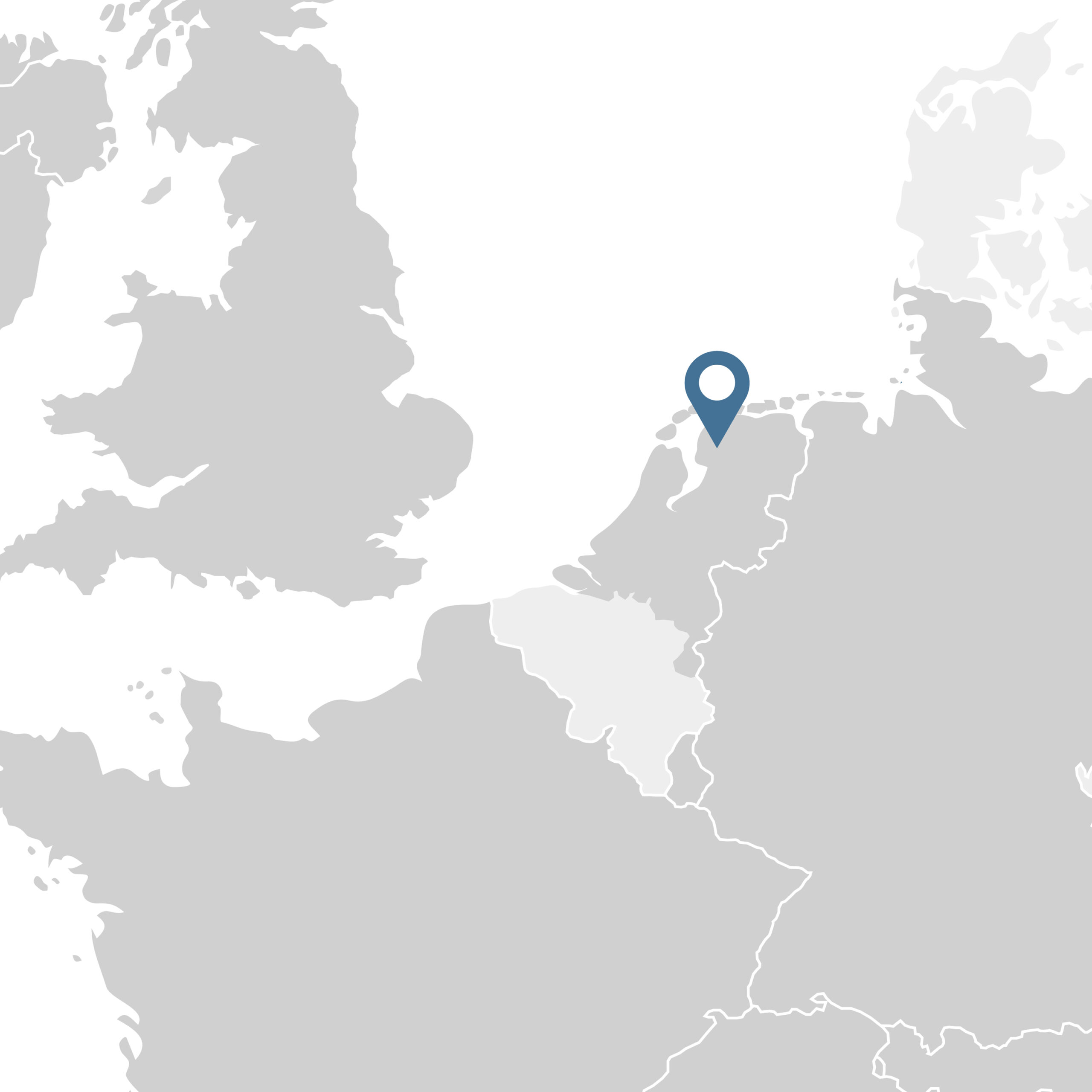
PCL production site, Drachten | Friesland, the Netherlands
Pilot Partners
Standards used
- OPC-UA
- Euromap 77/83
- JSON Header Body format (JBH)
Big Data Platforms & Tools
-
Azure Data Lake
-
Azure Time Series Insights
Big Data Characterization
Data Volume
57GB
Data Velocity
60MB/day
Data types
- Time-series,
- asset-data,
- Images,
- Operator logs
Number of sources
- Environmental data
- Quality measurements
- Raw material data
- Machine configurations
- Operational data
Open data
No
Dashboard & predicted IM parameter
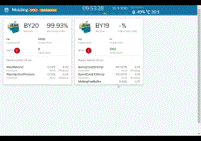