Big Data for Spare Part Predictive Warehouse Management
Whirlpool Trial: Better Spare Part Forecast powered by Machine Learning
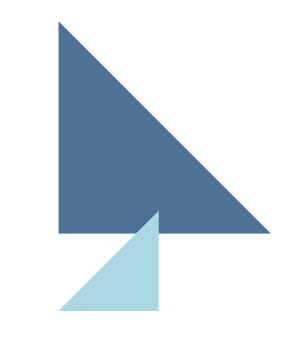
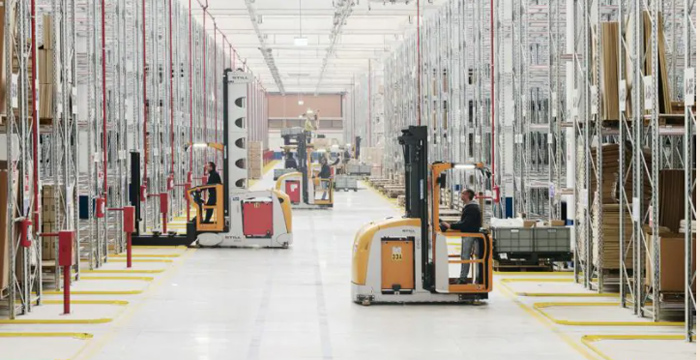
%
Demand Forecast Error Reduction
%
Human Effort for Planning Reduction
%
Spare Part Stock Value Reduction in terms of Value
%
Spare Part Stock Value Reduction in terms of Quantity
Data-Driven Digital Process Challenges
The management of spare parts in a multinational company which deals with huge numbers every day is a tricky challenge. The goal is to be ready at the right time with the right spare at the customer’s house and at the same time the challenge is maintaining the stock as low as possible.
Big Data Business Process Value
The Whirlpool Spare Parts Forecast pilot is concentrated in one main business process for implementation:
Part Forecast: The spare part production and management is one of the most complex and important challenges of a multinational company like Whirlpool, which is everyday dealing with a variety of suppliers, customers, and a huge number of different products and components. Considering the legal duty to keep parts for the all product lifetime of about 10 years and a rate of new product introduction every 18-24 months, the amount of different codes to be kept in warehouses is huge and so is the financial and economical effort to keep it working.
The quantity of parts to be kept available for field service is currently a compromise between the speed of satisfying the customers who wants their appliance repaired as shortly as possible and the financial and logistic capability to have that part available. This optimal solution, is achieved by forecasting the quantity of needed parts in each single market for a specified time period, with the objective of reducing as much as possible the amount and the value of the stock while keeping the availability.
Large Scale Trial Performance Results
A new forecast tool has been developed using Big Data dataset, characterized for high Variety and Volume: the historical data of spare parts consumption has been combined with Sales, Factory test, Device sell-in and out, Demand Planning and Service Calls.
The new tool proved to be much more effective in forecast accuracy and thus allowing to potentially reduce the spare part stock and increase the inventory turnover. Over than that, the tool is also much more efficient and the human overhead to review the data reconcile numbers and translate them into decision has been also lowered.
Observations & Lessons Learned
Despite large companies high availability of different data source, before BOOST4.0 was not so common to consider integration of such a high number of dataset and high volume of data. As a first result, WHR is now much more confident that the more data you can add on a decision support tool, the better the results. This is of course requiring some new technologies to be implemented and a increased consideration of accessories enabler such as High performance computing platforms, high capacity storage and common and uniform data modeling and interpretation.
Replication Potential
The first and immediate replication potentiality resides in other business process who rely on accurate forecast such as Finished Product Demand Planning (i.e. how the markets will require new products available in following year, months and weeks.
As a cascade, all the business processes related with planning could also benefit: translate demand into physical production plan combining factory and logistic capacity with multiple constraint to be considered (variability of production means, market campaigns, raw material prices and procurement etc.)
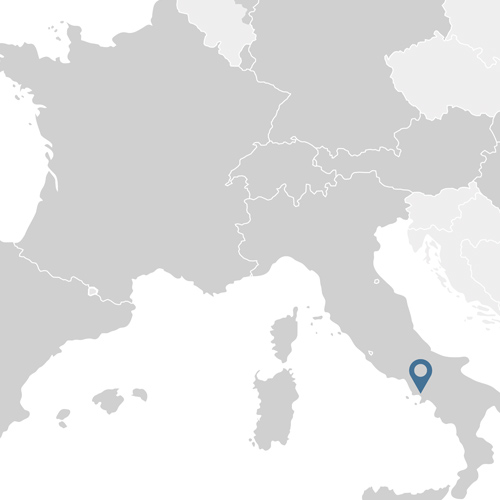
Whirlpool | Carinaro, Italy
Pilot Partners
Standards used
- Multiple Data Source
- SAP
- Standards Tables
Big Data Platforms & Tools
- SAS
- IMT
Big Data Characterization
Data Volume
25Mb per run
Data Velocity
Daily / Weekly
Number of sources
- Sales History
- Factory Test Data
- Device Sell-in / Sell-Out / Demand Forecast
- Service Calls
- Smart Appliances
Open data
No
Implementation Assessment
Technical feasibility
From technical point of view the solution is requiring a good data preparation and a solid technical partner
Economic feasibility
The ROI depends on the business process involved and on the implementation choice (SaaS, PaaS)
Replication potential
High replicable in similar business processes where forecast is required.