Autonomous Data Quality in the IDS
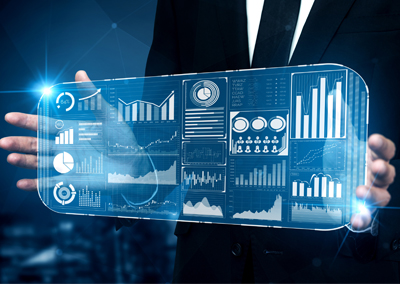
To reach the goal of Zero-Defect Manufacturing (ZDM) in light of complex industrial supply chains and information networks, we implemented a ‘Data App’ for autonomous data quality that is compatible with the International Data Space (IDS). This Data App ensures that high-quality data is shared with partners in a data ecosystem to avoid the propagation of erroneous data. We, hereby, cooperated with the Mondragon pilot for the design, implementation, and evaluation of our solution.
To determine the quality of the provided data set, we combined four different data quality measures that are suitable for industrial, sensor-based data streams and cover a variety of data quality dimensions. First, using an Isolation-Forest algorithm we perform an outlier analysis and calculate an outlier measure based on the commonness of outliers in the data stream. Second, a potential concept drift in the data stream is analysed on a per-block basis. This means that different data blocks are compared to determine whether predictions become inaccurate. The third and fourth data quality measures that we considered, are the ‘No Value Measure’, which looks for sensors that do not provide data over for a long time, and the ‘Constant Measure’, which detects sensors staying constant. These measures are combined to a uniform and easy-to-understand data quality score that is made available under the concepts of to the IDS information model and guidelines.