AIRBUS
Trade space framework for Autonomous Quality Manufacturing Systems’ Design
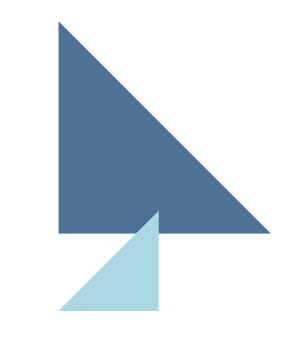
Airbus’ diverse product line includes everything from passenger jetliners to freighters and private jets. With each of the company’s aircraft family boasting cutting-edge design, superior comfort and unparalleled efficiency, they are setting the standard for the modern aviation industry.
Ranging in size from 100-seat jetliners to the highly fuel efficient A350 that is capable of transporting 400 passengers, the total versatility of Airbus’ product line allows it to offer a variety of tailored solutions to meet the needs of any airline and their market, from low-cost and full-service carriers to the air freight and VIP transport segments – while continuously ensuring the very best in efficiency and experience.
Process Challenges
Manufacturing an Aircraft needs to take into account all the challenges induced by the orders and the delivered Aircraft, but also the ones induced by new and future technologies of manufacturing (3D Printing, Hydro forming…) and the new manners of manufacturing (MBSE approach, Autonomous Quality, Digital Twins challenges, monitoring with IoT…).
During the early phase of an aircraft program, Industrial Architects are evaluating different industrial scenarios. One of the activity is to perform trade-off to optimize the future industrial architecture using different performance parameters like Labour cost, Industrial assets cost, Lead Time and different kind of risks within the extended enterprise.
Process Value
- An Industrial System Design will be demonstrated with an MBSE approach with co-design capabilities Industrial System to perform tradeoff in a more efficient manner.
- An interoperable prototype will be implemented, demonstrating seamless data flows in this process.
- Knowledge Capture, Management, inference will be demonstrated for a Knowledge-based (KBE) design approach.
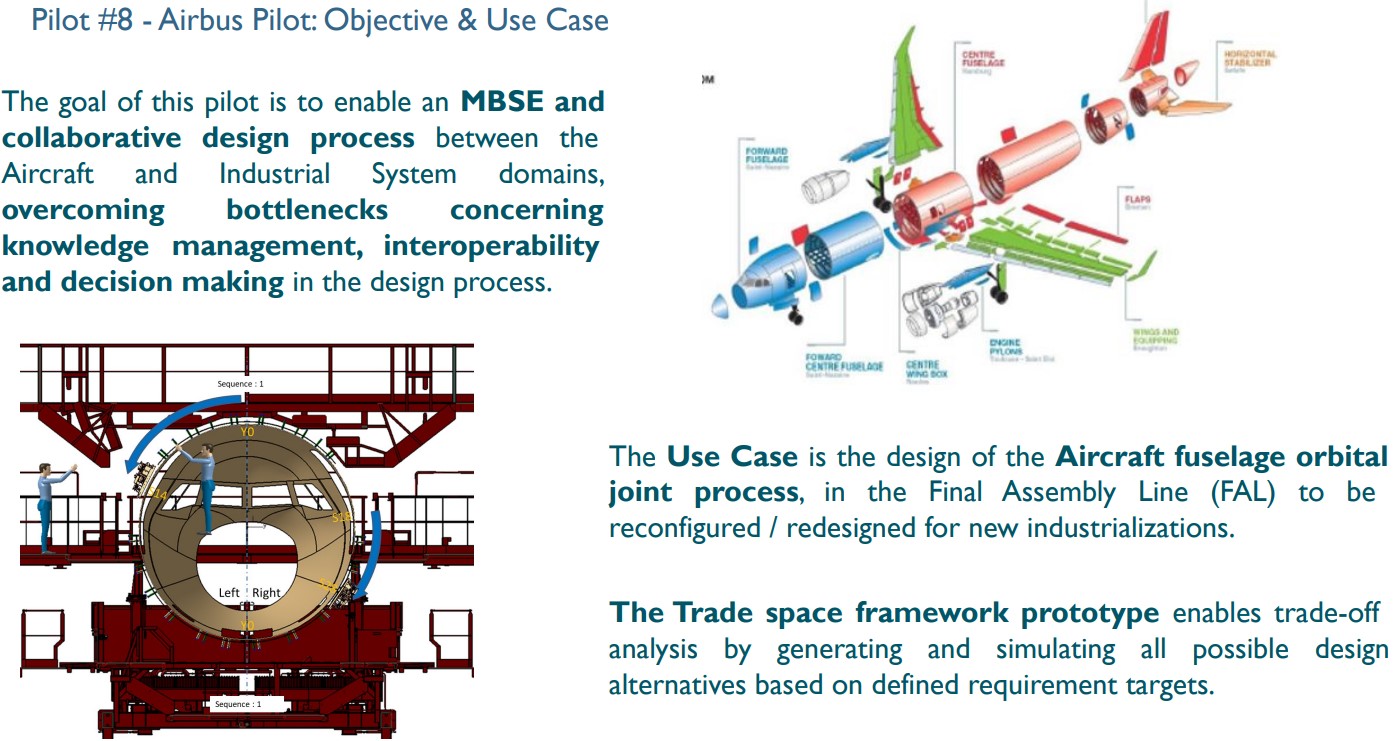
Experiment Performance
A demonstration of the Trade space study has been made with a major assembly process: the fuselage orbital joint of the A320 aircraft type in a New Final Assembly Line design. Among the trades performed are scenarios between a manual and a semi-automated process for drilling and riveting the orbital junction, with KPI’s of process time, throughput, OEE, and costs.
The Trade space framework demonstrated in this project, enables trade-off analysis by generating and simulating all possible design alternatives based on defined requirement targets. Its current maturity level is TRL5.
An application ontology was developed to capture domain knowledge, requirement and modelling knowledge. An automatic design solution generation was implemented based on querying and reasoning. Semantic integration of the dataflow was implemented, as well as ontology-based engineering principles for design automation.
The Trade space framework applies MBSE methods for Industrial System design, define system Requirements, generate Architecture models based on FLT approach and simulate the systems behaviour with Discrete-event and 3D space analysis simulation models.
Orbital joint process sequences can be automatically designed from previous experience, trading between possible scenarios. The framework is transferable to other manufacturing process design.
Value chain
Observations
1) This prototype has demonstrated how to standardize our interfaces so that they are predefined and are reusable. An application ontology was developed to capture domain knowledge, requirement and modelling knowledge, architected using domain independent ontologies.
2) The prototype developed shows concretely the digital continuity of a trade-off system using different domain models. Visualization is key to take decisions!
Lessons Learned
1) Technical – Ontology development is complex and may cause delays, need to optimize knowledge capture from domain experts & documents.
2) Implementation – Detailing of requirements model under consideration of integration and automation, plus need to make choices between a lots of design options.
Replication Potential
- It’s giving us good repeatability for our processes and additionally, it’s giving us a way to automate the boring parts of assessing all of the options that are resulting from our requirements in an automated manner.
- It helps on the analysis of the solutions generated, considering both configuration and lifecycle management. For next steps we need to consider resilience studies, and to foster the value of the 3D simulation in the loop.
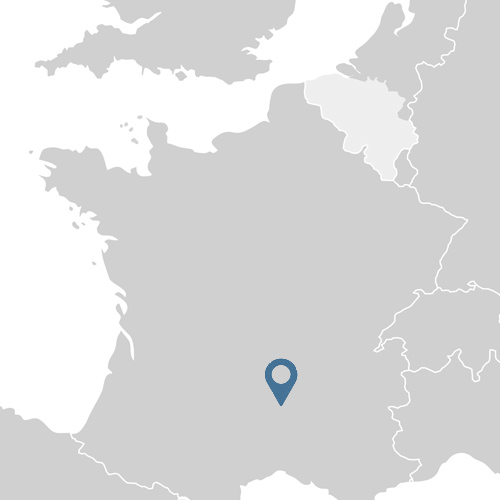
Airbus, France
Pilot Partners
Standards used
- CAD, Step (ISO 10303)
- Proprietary CATIA and VR datasets
- OWL v2
- RDF v1.1
Big Data Platforms & Tools
- OntReq
- MetaGraph
- SimPy & 3D simulation
- Neo4j + Azure
- Protege
Big Data Characterization
Data types
- Architecture models
- Requirements
- Discrete-event and 3D models
Number of sources
- 4 sources of information
Open data
No
Key Facts & KPIs
Productivity
PR1 – Production line performance
PR2 – Reduction of operator Load
PR3 – Working conditions & Labour Efficiency
PR4 – Reduction of production / manufacturing costs
Speed
SP1 – Ramp up time
SP2 – Trade-off Feasibility
Sustainability
SU1 – Human to machine ratio
Agility
AG1 – Lead time
AG2 – Time to market
Customization
CU1 – Flexibility for late customization
CU2 – Quality level
Adoption Assessment
Technical feasibility
Economic feasibility
Replication potential