Alum
Zero Defect Manufacturing for Aluminum Extrusion Industry QU4LITY_ALUM
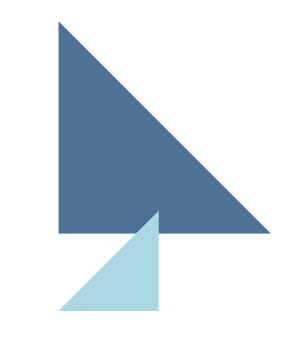
EXALCO is an integrated industrial unit producing aluminum profiles, having the possibility to cover all the modern structural and architectural needs. All the people of EXALCO are focused to the continuous progress and the development of the services rendered, offering quality products with respect and sensitivity towards the natural and social environment. Nowadays, EXALCO has developed an extended sales network in domestic and foreign markets and holds justly the leading position in the field of Greek aluminum extrusion.
Process Challenges
Quality assurance is crucial in the Aluminum Extrusion Industry and the quality requirements are highly demanding. There is a clear need to optimize the quality process and the management of the quality issues at plant level and drive the traditional methods (i.e. currently off-line and not at work-cell level) towards development. Therefore, the use and integration of new technologies, (Artificial Intelligence) in the production process is imperative to tackle the market and environmental demands and achieve the Zero-Defect Manufacturing concept.
Process Value
The EXALCO ZDM project focuses in developing a tool to predict the unusual behavior of the gasket in the press, caused by degradation, which can lead to lower quality standards. The integration of new sensors in the equipment in combination with the already existing methods is the first part of the solution. The main innovation is the use of new technologies such as Artificial Intelligence which will exploit the data and discover trends that will lead to the machine anomalous behavior. The system through real-time data analysis will provide information about the status of the machine directly to the user supporting the aluminum extrusion process.
The QU4LITY_ALUM solution exploits different sources of data to identify the trends of the equipment that can lead to failure and indicates the remaining useful life of the component.
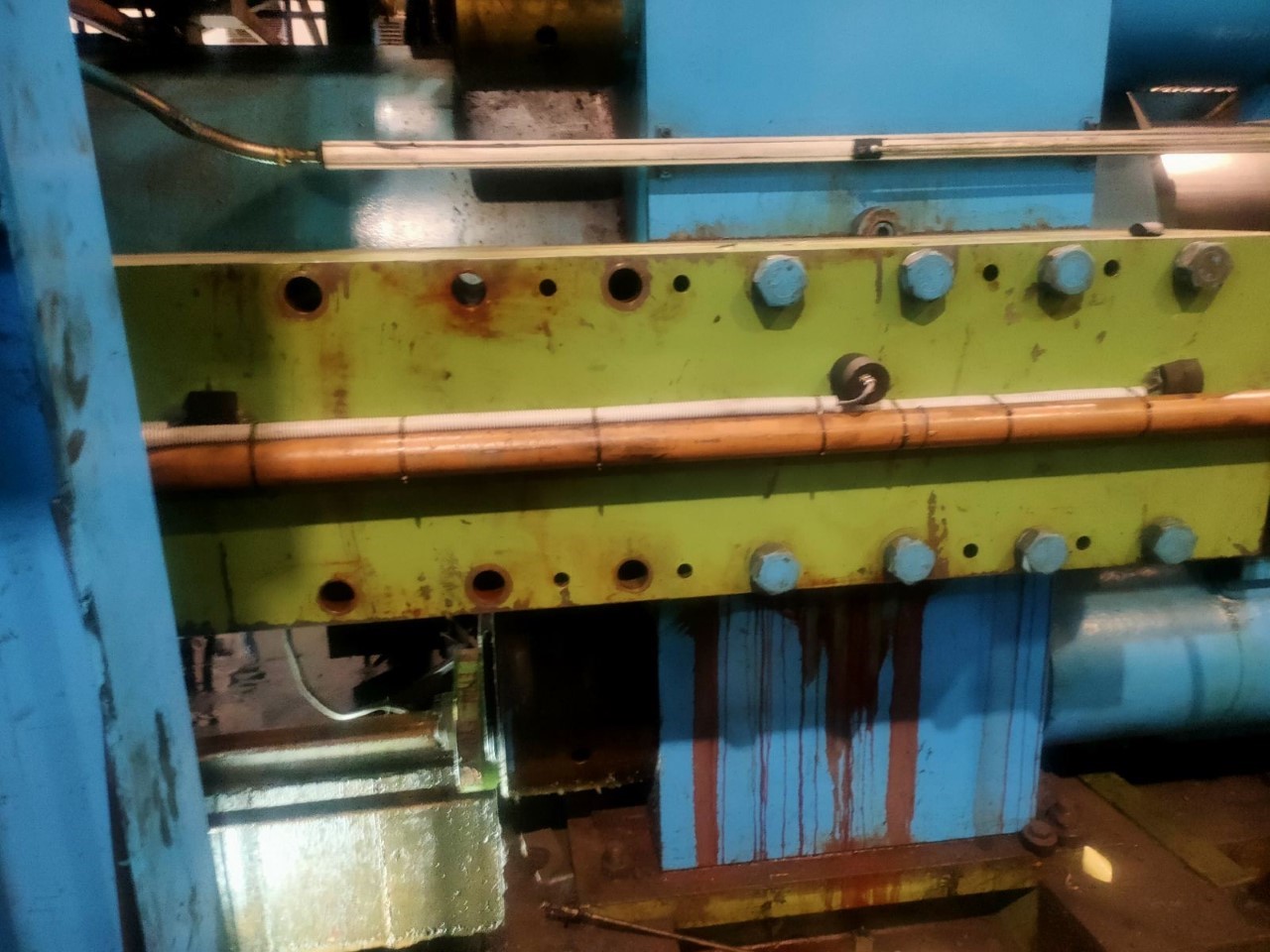
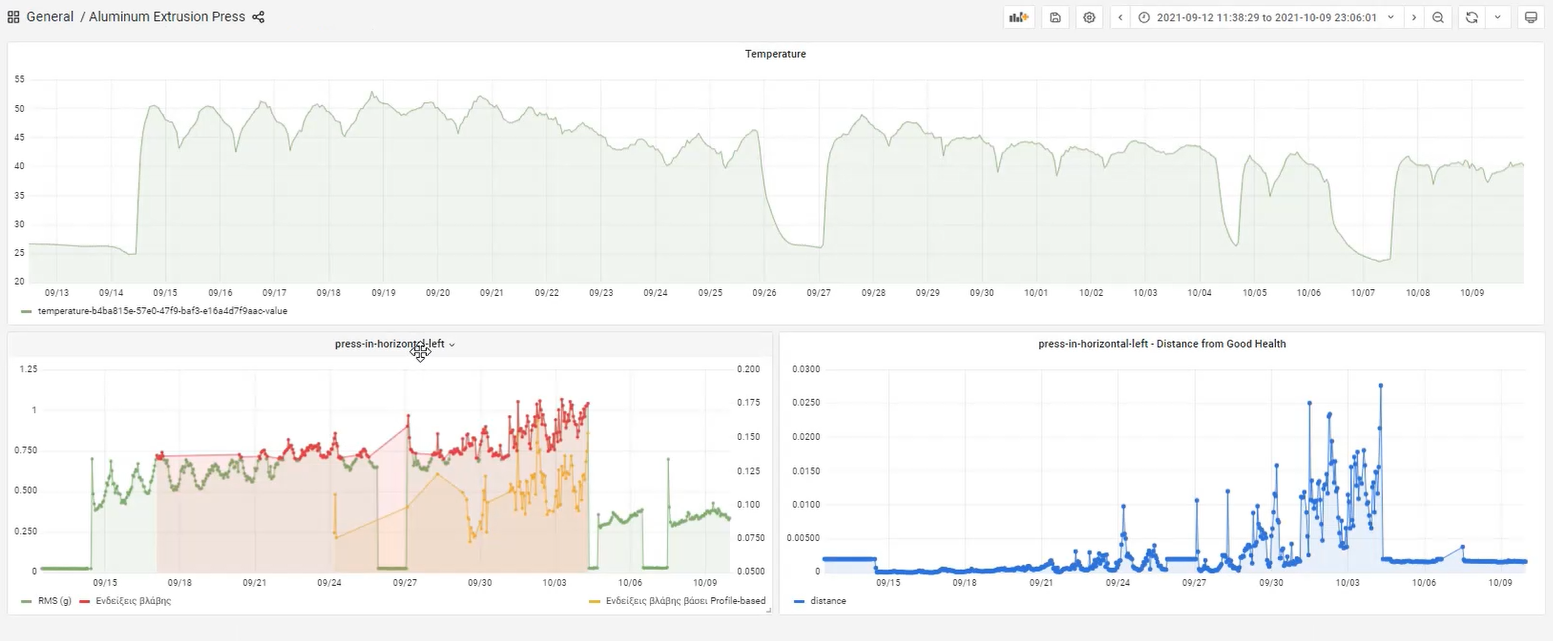
Experiment Performance
The EXALCO trial has aimed in developing a RUL enabler that will exploit artificial intelligence and the data from multiple sources to estimate the remaining useful life of the equipment.
Using machine learning models and a large quantity of different data the tool has identified the trends existing in normal equipment function. The continuation of the experiment after the end of the project will provide the necessary inputs to estimate the remaining useful life.
These information that can be provided through the solution have allowed an enhanced machine monitoring, which is important to keep the product quality high. In parallel, through the data analysis, the user can schedule timely maintenance activities, decreasing machine downtime and the environmental footprint as well as increasing the worker’s safety.
Value chain
Observations & Lessons learnt
This approach can showcase the necessity of combining the technology with the equipment and the success factor can be attributed to the close cooperation between the technical providers and field experts.
As a result, valuable information can lead to crucial improvements. Artificial intelligence, machine learning and the integration of sensors can support the production and the timely maintenance scheduling by detecting and predicting the degradation of equipment. The main benefit of the solution is that it exploits real-time data from different sources efficiently to monitor the machines. This tool can lead the factories in the digital transformation era and the maintenance systems from detective to predictive. The user will receive timely information to actively resolve and prevent the issue ahead of time, ensuring the smooth continuation of the operations.
Replication Potential
The solution is data-driven and with the right parametrization can be applied in a variety of scenarios and in different fields. Especially, the solution can be deployed easily in testing environments and the data transfer is reliable and fast. The solution integration is swift and the complex machine learning models can achieve the same reliability and accuracy with the necessary specifications in different types of equipment.
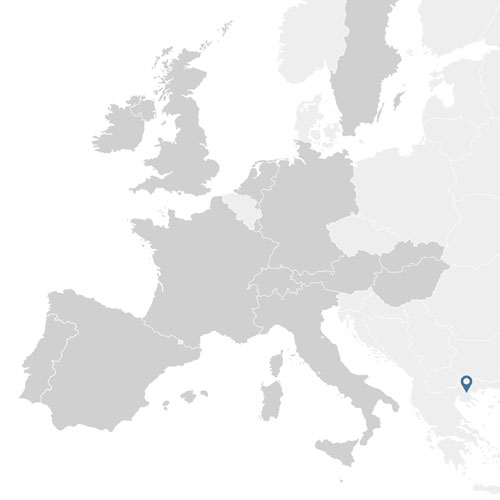
Thessaloniki
Pilot Partners
Standards used
- JSON
- MQTT
Digital Platforms & Tools
- Proprietary Platform
Big Data Characterization
Data types
- Temperature
- Ultra-sound
- Pressure, speed and position of the Ram
Number of sources
3 sources
- Temperature sensors
- Ultra-sound sensors
- PLC
Open data
No
Key Facts & KPIs
Productivity
Quality yield 99% -> 99,2%
First Pass Yield 99% -> 99,2%
Asset life extension 1 y -> 2 y
Maintenance and operational costs 17.000 -> 15.000€
Speed
Machine Downtime 248h/y -> 200h/y
Sustainability
Production line availability 82% -> 85%
Overall equipment effectiveness 65% -> 67%
Agility
MTBF 9d -> 10d
Adoption Assessment
Technical feasibility
Economic feasibility
Replication potential
RUL enabler provides a set of data driven micro-services for the Remaining Useful Lifetime (RUL) estimation and the Fault Detection. Supporting micro-services for pre-processing the data are also used to clean and transform the data to compatible forms. All these micro-services are mapped to the Factory layer and more specifically to the “AI/ML Training Analytics and Data-driven Services”. The enabler also offers visualization of the collected sensorial measurements and the computed from the analysis results. The visualization component of the solution is mapped to the factory layer and more specifically to the “Simulation and Visualization Services”.